As AI continues to make waves across industries, IT leaders must ensure that the vendors they choose to do business with have a responsible, ethical AI approach.
The increasing impact of ethical AI is of critical importance to IT decision-makers looking to better understand and implement transparent systems and practices. This article includes the following key takeaways:
- AI is rapidly being integrated into software like intranet platforms, bringing risks like bias and privacy violations. This makes ethical AI critically important.
- Ethical AI involves principles like fairness, accountability, transparency and respect for privacy and consent. It ensures AI doesn’t unfairly discriminate or erode trust.
- IT leaders evaluating SaaS vendors should thoroughly vet their ethical AI practices around data handling, algorithm transparency in AI, governance and more.
- Asking probing questions about issues like bias testing, model explainability and accountability controls is key to assessing vendor maturity.
- Ethical AI mitigates risks, builds employee productivity and trust, and sustains corporate culture and values. It should be a top priority.
- 1 The rise of AI and the importance of ethics
- 2 What exactly is ethical AI?
- 3 Why ethical AI should be on every IT leader’s radar
- 4 Key considerations for AI ethical evaluation in software development
- 5 Vetting vendors: 12 AI ethics questions for IT decision-makers
- 6 Simpplr: Where employee experience meets ethical AI
- 7 Frequently asked questions
The rise of AI and the importance of ethics
Artificial intelligence (AI) is rapidly revolutionizing software development. Gartner predicts that by 2026, 80% of new enterprise apps will use AI. This reflects an annual growth rate of 37% reported by Grand View Research (via Forbes) between 2023 and 2030. However, as these advanced systems proliferate, so do their potential downsides — like encoded biases and privacy violations.
In response, more organizations are recognizing the role ethical AI evaluation should have in their selection of IT solutions.
From ensuring transparency in AI to defining and implementing responsible AI across business systems, ethical AI is becoming a front and center issue for IT leaders.
Notably, ethical AI is not solely a concern for the software industry. According to Statista, ethical AI is rising in importance across industries: “In 2021, industrial manufacturers appear to be the quickest industry to adopt ethics policies for artificial intelligence (AI), with 89 percent of respondents from the manufacturing industry indicating that they already have such a policy within their organization. Other industries that are quick to implement ethical AI policies include retail and technology.”
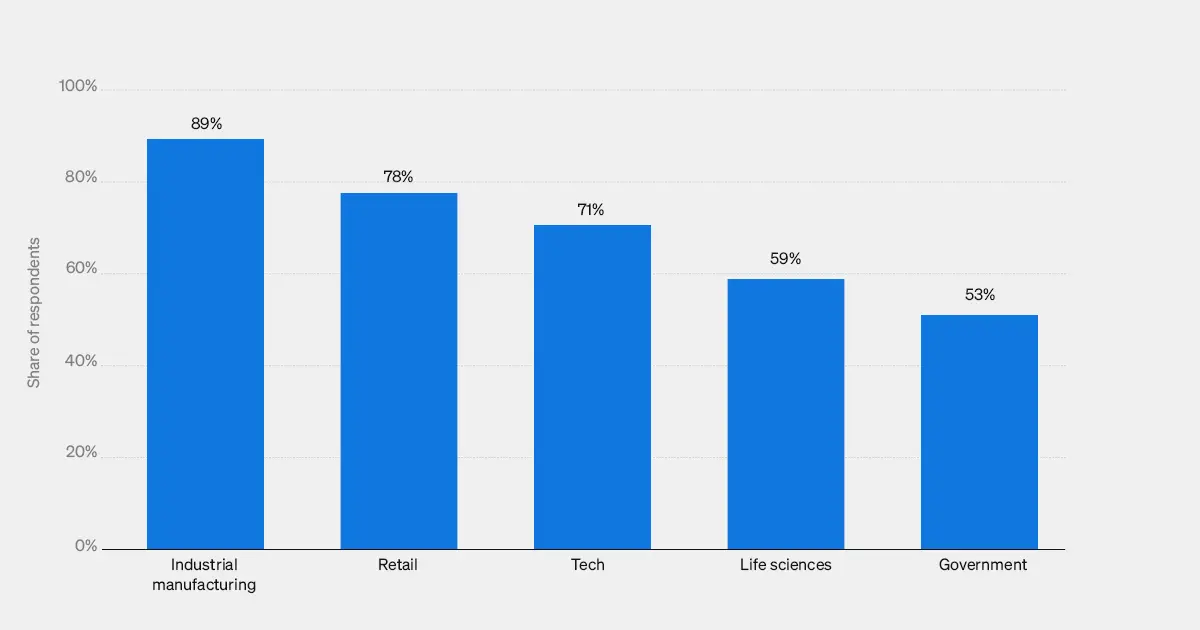
This makes ethical use of AI critical for vendors building software with access to sensitive user data. For organizations evaluating SaaS solutions like intranet platforms, scrutinizing AI ethics should now be standard practice. Here’s why:
- Mitigate business risks: Ethical AI can prevent issues regarding bias, discrimination and surveillance that can create openings for discrepancies, and even litigation, down the road.
- Build employee trust: Outlining responsible AI practices can demonstrate an organization’s dedication to privacy and fair treatment.
- Safeguard company culture: Transparency in AI used within intranets can enhance engagement while keeping inclusion intact.
What exactly is ethical AI?
Ethical AI, or AI ethical evaluation, ensures technologies do not unfairly discriminate, breach confidentiality, violate consent rights or otherwise contravene core moral values.
The practice requires transparency so AI systems are interpretable by external auditors, evaluating for harms like bias against minority groups or workers with disabilities.
Vendors must then be accountable for correcting issues discovered.
Ethical AI should not be considered optional in spaces like employee intranets that handle a large volume of corporate data; it’s paramount in order to avoid ethical breaches that erode workplace culture.
It’s already risky having workplace data extracted and crunched every which way. When adding AI algorithms to the mix, guardrails are definitely needed.
That’s where ethical AI comes into play. Deciding to conduct an AI ethical evaluation means designing and managing AI in a way that respects end-user privacy while avoiding unintended harm.
Some of the key areas included in ethical AI are:
- Fairness: Making sure predictive models don’t discriminate based on race, age, gender identity and other protected categories.
- Explainability: Requiring data scientists to account for how models work to cultivate transparency.
- Accountability: Addressing issues as they occur in an equitable manner.
Why ethical AI should be on every IT leader’s radar
AI is becoming more prevalent across intranet platforms promising smarter search, personalized recommendations and even chatbots acting as virtual assistants.
But for those responsible for vetting new software that touches vast amounts of employee data, there can be warning signs during the evaluation process that many leaders new to AI ethics may overlook.
Keep in mind that AI in the wrong hands can introduce risk and adverse outcomes that can cause harm to individuals and organizations alike, even those with the best intentions.
When exploring solutions, IT leaders should pay specific attention to whether a software vendor becomes uneasy or evasive when answering questions about ethical AI practices. It takes rigorous testing, auditing and governance to establish ethical AI in intranet software development, and prospective customers need a clear understanding of everything from safety and security to data protection regulations to ensure that the vendor they choose is in line with best practices.
Key considerations for AI ethical evaluation in software development
As AI becomes further integrated with intranet platforms, powering features like personalized recommendations and intelligent search, it promises to revolutionize the employee experience. However, without an ethical framework guiding AI implementation, these tools risk amplifying biases, eroding privacy and undermining trust.
Intranet software vendors must bake core ethical principles into their AI systems from the start.
These areas encompass transparency, accountability, equity and privacy.
Transparency in AI
AI explainability is key for intranet systems that customize recommendations and surface content to employees. The logic behind suggestions or matched profiles should be human-interpretable.
For example, natural language processing algorithms that analyze employee skills and interests to recommend potential mentors should be transparent on the attributes weighted in the matching criteria. If they consider sensitive factors like gender or ethnicity without disclosure, hidden biases are enabled.
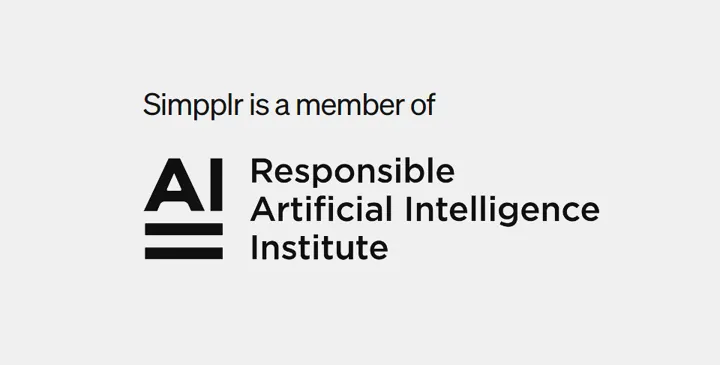
Simpplr’s intranet platform is accompanied by documentation on model functionality so clients understand how outputs are determined. Simpplr is also part of the Responsible Artificial Intelligence Institute, which is dedicated to driving trustworthy AI.
Accountability in AI
Vendors must implement checks and balances to regularly audit AI systems, identify emerging risks from new data or use cases and correct issues. Accountability in AI should be focused on including safeguards that will protect user data from intentional and unintentional misuse by end users and the AI itself.
One survey produced in partnership with the University of Oxford showed that over 30% of IT decision-makers had no formal process to preemptively assess AI harms.
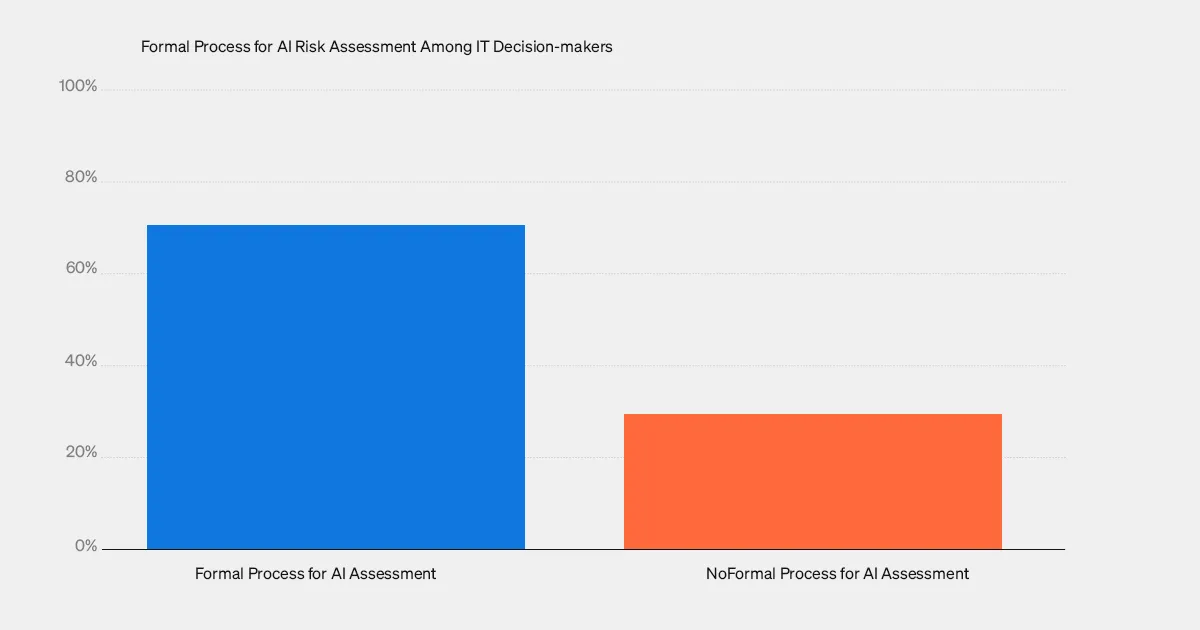
Once an organization establishes processes to monitor and identify problematic issues, IT leaders should then implement policies that outline exact responsibility for ongoing governance. These steps often include:
- Appointing responsible AI oversight teams who continually assess for fairness and other issues.
Conducting impact assessments before launching new AI features. - Providing secure channels for employees to report problems like irrelevant content management.
Establishing procedures to promptly remove underperforming models.
Fairness in AI
From performance reviews to automated recruitment filtering, AI risks perpetuating biases that discriminate against minorities, women, older staff and people with disabilities. Fairness in AI applies to the practice of solving for potential prejudice that could result from training algorithms, data sets and human intervention. In contrast, a bias is a decision-making component that results in unfair or inequitable outcomes.
Avoiding bias in AI starts with the training data used to establish the system’s practices.
In the case of intranet platforms, this data must represent workforce diversity, equity and inclusion (DEI). Other factors include data collection and user interaction.
It’s important to understand that evaluating model outputs alone is insufficient. IT leaders and system administrators should also be able to review and contest AI decisions that reflect unfair practices or perpetuate discrimination. This can be especially critical to intranet platforms designed, in part, to provide a diverse and fair space for employees to engage with one another and the company.
Privacy and consent in AI
With the threat of data breaches at major corporations becoming what seems to be a common occurrence, securing employee information that feeds intranet AI is non-negotiable. Vendors must allow custom permissions, access controls and opt-out preferences rather than mandating blanket data collection.
Transparent policies explain what is captured, why it’s needed, and how it is protected.
These guidelines also clearly establish how data is disseminated so users understand exactly how their information is being used by the company and any other applicable third parties. Authentication safeguards and auditable access trails prevent exposure through insider threats as well.
Weaving these principles into the AI development lifecycle fortifies employee engagement and trust. It also mitigates severe downside risks for vendors like damaged credibility or legal liabilities from failing to be responsible AI custodians.
Discover strategies to reduce software supply chain risks
Vetting vendors: 12 AI ethics questions for IT decision-makers
AI promises to amplify productivity and efficiency across nearly all enterprise software, especially in the case of intranet platforms. Digital workspaces stand to revolutionize how employees engage with one another and the organization while providing organizations with essential data analysis that can streamline human resources, internal communications and other processes.
Without governance, irresponsible AI design by SaaS vendors places your data, employees and company at risk.
IT decision-makers evaluating potential solutions to be entrusted with sensitive information should make ethical AI a top priority. Here are 12 questions to guide your conversations as you thoroughly assess vendor maturity.
Data practices
- What specific employee or customer data feeds into your AI systems? Do individuals have control through permissions or consent preferences?
- How is personal and behavioral data protected through de-identification, access controls, encryption and other safeguards? What assurances or certifications do you have around data security?
- What rights and choices do we have around data transparency in AI, portability to other systems and deletion requests? Can employee data use be opted out of?
Algorithm transparency
- Are your AI models interpretable, meaning the workings and logic behind outputs can be understood? Can documentation be provided to support this?
- How do you choose training data that represents diversity across all protected classes such as race, gender, age and disability status?
- What techniques or constraints ensure your models operate fairly without unlawful discrimination or inherent biases? How is this validated?
Governance
- What internal roles and oversight processes are in place to continually assess your AI systems for harm? How rapidly can issues be flagged and addressed?
- Does your organization have a formal code of ethics for AI practices ingrained across teams like data science and engineering?
- Are external audits conducted on the ethical use of AI? Who performs these audits and how frequently?
Regulatory compliance
- Which specific governmental regulations, industry standards or internal policies guide your governance of AI?
- How could we be notified of any AI incidents, data breaches or regulatory non-compliance from your organization?
- What liability protections or remedy processes protect customers and their data subjects in case of disputes, violations or harm?
Pro tip: SaaS vendors handling sensitive data are stewards of public trust. Vet them thoroughly as a gatekeeper against irresponsible AI.
Simpplr: Where employee experience meets ethical AI
At Simpplr, artificial intelligence continues to shape our intranet platform with a strong focus on ethical AI governance. Our human-centric approach to ethical AI in intranet software development focuses squarely on amplifying each employee’s potential through thoughtful, ethical design.
We incorporate AI as an intuitive assistant, not just flashy automation. Features like My Team Dashboard analyze usage patterns and sentiment signals to guide managers in better supporting their reports insight by insight, not as robotic directives.
Our virtual assistant answers employee questions with human nuance. The goal is always to augment individual judgment, not supplant it.
Unlike many vendors making the same claims but rushing half-baked AI to market, we build ethical practices into our AI in the software development lifecycle from the start.
Accountability over automation
While AI optimizes parts of our platform to better serve users, Simpplr remains accountable for governing risks. We continually audit for emerging harms, enacting fixes swiftly as issues arise.
Our leadership in ethical AI has captured the industry’s attention. As Gartner® noted in their 2023 Critical Capabilities Report, Simpplr fuses AI throughout functions from analytics to personalization and surface insights for both employees and intranet managers. But our technology stays focused on enhancing human choice, not restricting it.
Consent drives data
We prioritize the minimal collection of necessary employee data for our algorithms, ensuring that individual rights guide what information is gathered. This approach builds trust in the responsible utilization of data, emphasizing our commitment to data privacy.
Explainable and fair
Our AI model documentation details how outputs and recommendations are determined so they stay understandable and equitable. Rigorous testing removes biases that could disproportionately impact marginalized employee groups.
Request a demo to see firsthand how Simpplr leverages the power of responsible AI to enhance and elevate employee experiences while boosting productivity.
Frequently asked questions
What are the main ethical principles companies should follow for AI?
The big five pillars tend to be transparency (clear explainability into model logic), fairness (preventing discriminatory outcomes), accountability (auditing for issues), reliability (technically solid) and privacy/security (data protections). Adhering to these values from the initial design stages helps AI earn public trust.
What risks typically worry people when it comes to AI systems?
Understandably, AI risks have received recent media attention especially regarding the threat of unfair bias and the impact of technology on human-centric jobs. But issues like transparency, manipulation and surveillance that lack human oversight also rank high among public concerns. Proactively self-governing and partnering cross-functionally help mitigate these downsides.
What ethical problems can AI content generators introduce if not developed responsibly?
It’s important to note that AI content generators sometimes generate responses that are not properly aligned with the prompts they receive, which can cause issues around privacy policy violations, security exploits, spreading misinformation, plagiarism or toxic speech, and perpetuating unfair societal biases. Continually assessing outputs and being able to explain why models make certain choices is crucial to keep them behaving ethically.
How do we know AI won’t cost people jobs?
Historic transitions like automation have often displaced jobs even while raising productivity. The key with AI implementation is proactive collaboration between technologists, policymakers and community advocates to make reskilling and job transition resources available.
AI should augment human strengths, not subsume livelihoods. We’re all responsible for sustainable progress.